Yankees: How can we analyze Giancarlo Stanton differently?
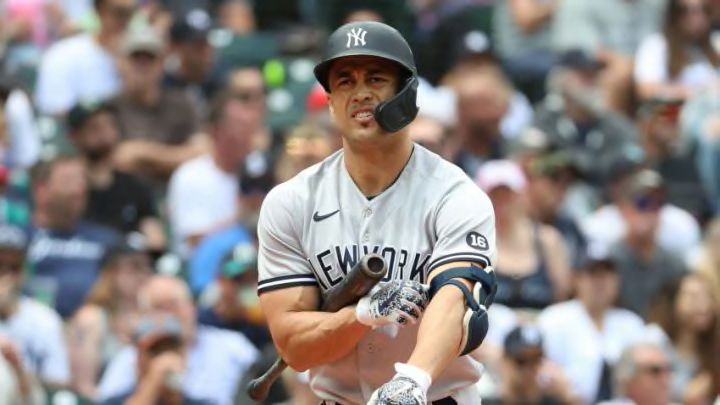
Listening to a recent episode of the Yanks go Yard podcast, I found myself conflicted. Hosts Adam Weinrib and Thomas Carannante were frustrated with Yankees’ designated hitter Giancarlo Stanton. But they were also frustrated with some of Stanton’s defenders.
For the “pro-Stanton” camp, the slugger deserves his dues for his overall solid offensive numbers (as I write this he has a 120 OPS+). But as Weinrib and Carannante were quick to point out, those numbers don’t tell the whole story. Stanton is a player who can go on impressive hot streaks, driving up his numbers. But he also spends significant portions of his playing time struggling to even make contact.
This criticism of Stanton makes sense. If a “good player” is not good all the time, then you can’t count on them when it matters most: in close games, with runners in scoring position, in the playoffs, etc.
Alternatively, think of it this way. Imagine you’re in school, and one of your classmates is an A+ student who always procrastinates until the last minute. Would you rather do a group project with a less impressive, but consistent and responsible student, or take on that A+ student and risk that this time she might take her procrastinating ways too far?
So I agree that a player’s talent has to be weighed against their consistency. Nonetheless, I had a problem with how the Yanks Go Yard hosts raised this argument. The hosts argued that a certain generation of fans relies too much on stats, and thus misses out on what happens when the game is actually played.
This kind of rhetoric is all too common in baseball’s contemporary culture war, with some going so far as to define themselves as “pro” or “anti” analytics. “Analytics” just means the interpretation of data. The debate should not be about if we analyze data, it should be about how we do it.
Stumbling through the consistency data when it comes to Giancarlo Stanton
I decided to compare three Yankees for consistency. I looked at Giancarlo Stanton, Aaron Judge and Gio Urshela. I chose Judge because he’s viewed as a similar type of player to Stanton, but more reliable. And I chose Urshela, because he is often hailed by broadcasters John Sterling and Suzyn Waldman as “the most consistent Yankee.”
Unfortunately, comparing these players without a thorough statistical education (and a lack of access to relevant software) proved a frustrating task. Stanton spent much of 2019 and 2020 injured. Judge has also lost substantial playing time to injury. And all three players started playing for the Yankees in different seasons — 2016 (Judge), 2018 (Stanton) and 2019 (Urshela).
Joey Gallo has only grounded into 9 double plays in his career
— Talkin' Yanks (@TalkinYanks) July 29, 2021
Giancarlo Stanton, Gio Urshela, Gary Sanchez, Aaron Judge and DJ LeMahieu have all grounded into 9+ double plays this season
With this in mind, I decided to take data from this season, and combine it with data from each player’s other healthy year with the Yankees. That way I could see whether, in years when the players “felt like themselves,” they performed consistently or not. So for Stanton, I looked at stats from 2018 and ’21, for Judge from 2017 and ’21, and for Urshela from 2019 and ’21.
I took the players’ batting averages, on-base percentages and slugging percentages from each month (so roughly 10 months worth of each statistic for each player), and calculated the standard deviation (a measure of statistical consistency) for each metric.
Here’s what I found. The standard deviations for Stanton (in the order: batting average/on-base percentage/slugging percentage) were 36.4/38/78.6. The standard deviations for Urshela were 56.6/53.3/114.8. The standard deviations for Judge were 49.3/60.4/161.4.
In other words, by this measure, Stanton is the most consistent player of the three (a lower standard deviation means more consistency)!
Data analysis
So what does one take from this data? One lesson is to avoid the trap of anecdotal thinking. Urshela seems consistent because he was acquired by the Yankees as a bench player. The fact that he consistently outplays those initial expectations makes him far easier to be impressed by, than the high-paid, former MVP, Stanton.
Giancarlo Stanton has a .354 slugging pct in his last 55 games (since May 7).
— Katie Sharp (@ktsharp) July 31, 2021
That's his worst slugging pct in any 55-game span in his career.
Another important nuance is that consistency is a stat that has to be looked at in context. Judge slugged an other-worldly .889 in September of 2017. Just because he doesn’t consistently hit that high, does not mean he has a meaningful consistency problem.
A third takeaway from this data works less in Stanton’s favor, however.
For all three players, slugging percentage was by far the least consistent statistic. Stanton’s standard deviation for batting average was 36.4, whereas for slugging percentage it was 78.6. Urshela’s standard deviation for batting average was 56.6 and for slugging percentage was 114.8. Judge’s standard deviation for batting average was 49.3 and for slugging percentage was 161.4.
In short, no matter whether you’re a scrappy infielder, or a brawny DH, slugging is a particularly hard skill to pull off with consistency.
So what do we make of Yankees slugger Giancarlo Stanton?
One of the lessons of my little trip into data-land is that statistical analysis really is a field that requires expertise. A more baseball-specific form of standard deviation would have to figure out over what units of time to calculate player consistency (I used months, but not every player is equally active in each month).
It would also have to account for league-wide differences in statistics that aren’t indicative of a player’s ability (comparing batting averages between 2001 and 2003, isn’t the same as comparing them between 2019 and 2021, as many players spent early 2021 batting below .200).
Most 110+ mph HR tracked by Statcast (since 2015):
— Sarah Langs (@SlangsOnSports) July 29, 2021
Giancarlo Stanton: 94
Nelson Cruz: 71
Joey Gallo: 52
Aaron Judge: 47
Mike Trout: 47
But even my little study shows the complexities of what it means to evaluate a player and build a roster. As far as MLB players go, Stanton may still be a top-tier talent, and he might be just as consistent as anyone. But if all power-hitting is necessarily inconsistent, than perhaps the type of player Stanton is, is simply not one the Yankees need right now. If you’re going to be an inconsistent slugger, your home run rate has to be more like Shohei Ohtani’s than like Stanton’s.
Then again, well-timed inconsistency can be a good thing. Stanton has a .606 career slugging percentage in August and .519 career slugging percentage for September. Let’s hope the summer heat unlocks his much needed power!