Sabermetric Outlook Series: A Primer
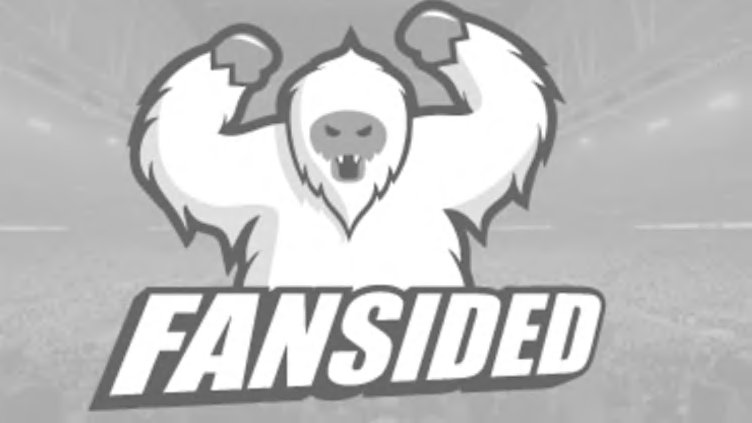
In the following weeks/months, I’ll be writing a series of “Sabermetric Outlook” posts. Each post will look at a specific player on the New York Yankees, starting with the infield, then moving to the outfield, starting pitching, bullpen, and bench.
My goal in these posts is two-fold; first, I want to provide an analysis of some of the more advanced statistics of the Yankees’ players, both to evaluate their performance thus far and predict how they will fare in the future.
Secondly, I hope that these posts can be used as a useful introduction to sabermetrics for those who are used to more traditional stats, and will give readers a starting point for using advanced statistics to evaluate players. I also welcome any and all feedback and discussion about these posts and the players involved.
For this first post, I’m going to provide a primer of some of the most important stats and principles I’ll be using to evaluate players. Obviously I can’t list all of the stats that I’ll use here, but hopefully this will be a helpful starting point for those unfamiliar with sabermetrics, as well as a guide to refer back to in future posts.
Offense
OPS (On-Base Plus Slugging): OPS is a pretty simple stat. It is the sum of a player’s On-Base Percentage (OBP) and Slugging Percentage (SLG), thus combining singles, walks, and extra-base hits all into one simple metric. OPS is used by many as a starting point (and sometimes ending point) for measuring offensive performance. In 2011, the average OPS was .720, and the leader was Jose Bautista with a 1.056 OPS.
wOBA (Weighted On-Base Average): wOBA measures basically the same thing as OPS – contact, patience, and power – but assigns different amounts of weight to the various outcomes. For example, while homeruns are 4 times as valuable as singles in OPS, they are only about 2.3 times as valuable as singles in wOBA. These weights are based on how many runs each outcome is normally worth in the course of the game. I won’t get into more detail than this, but if you want to read more about how wOBA is calculated, I suggest checking out The Book by Tom Tango, who invented wOBA.
wOBA is set to about the same scale as On-Base Percentage, so league average in 2011 was .316, and the leader was again Bautista with a .441 wOBA. In the course of this series of posts, I will primarily use wOBA instead of OPS, as it more accurately measures the importance of each offensive outcome.
wRC+ (Weighted Runs Created Plus): This is my personal favorite sabermetric stat. Basically, it uses a player’s wOBA to determine how many runs the player created (a single is worth about .46 runs, a double .75, and so on), then compares that number to league average while also adjusting for the ballpark. It is scaled so that 100 is league average, and every point above or below 100 is one percentage point above or below league average. In other words, if a player has a wRC+ of 140, then they are 40% better offensively than the average player. Alternatively, if a player has a wRC+ of 90, then they are 10% worse than the average player. Last year, the leader in wRC+ was (surprise surprise!) Bautista with 181.
I will probably use wRC+ more than any other sabermetric stat in this series, as it measures all-around offensive performance, adjusts for the league and park, and very quickly shows how a player relates to the rest of the league.
BABIP (Batting Average on Balls in Play): BABIP is exactly what it sounds like; it is a player’s batting average taking into account only balls that are put in play (minus home runs). In other words, it doesn’t care about strikeouts, walks, hit-by-pitches, or home runs, and only measures how often batted balls that stay in the park fall in for hits.
There are a lot of misconceptions about BABIP, one of the major ones being that a hitter’s BABIP is completely based on luck. This is absolutely not true. Although BABIP is often strongly influenced by luck, there are wide range of expected BABIPs based on a player’s speed, batted ball profile (ratio of groundballs to flyballs, for example), and other factors. Ichiro Suzuki, for example, has a career .348 BABIP, while Carlos Pena has a career .277 BABIP. These differences are not due to back luck, but to the drastically different hitting styles of Ichiro and Pena.
That being said, batters can still have BABIPs that are far different than what is expected based on their speed and their batted ball profile. In these cases, we can often attribute this difference to good or bad luck, though further investigation into the reasons for this is always necessary. For reference, the league average BABIP last year was .295.
Plate Discipline: This is not a specific metric, but a group of stats that tell us about a hitter’s patience, contact, and batting eye. I won’t go over every specific metric here, but it is important to know that whenever the stat starts with Z, it is dealing only with pitches inside the strike zone, and when it starts with O, it deals with pitches outside the strike zone. For example, Z-Swing% is the percentage of pitches inside the strike zone that a batter swings at, and O-Swing% is the percentage of pitches outside the strike zone that a hitter swings at.
Pitching
FIP (Fielding Independent Pitching): FIP is actually very simple; it takes a pitcher’s strikeouts per 9, walks per 9, and home runs per 9, and calculates what that pitcher’s ERA should be given an average BABIP (see above or below) and timing of outcomes. In other words, it takes the aspects of pitching that are independent of the pitcher’s defense and are most in the control of the pitcher. While this is not perfect way to measure a pitcher’s skill, it does, for the most part, measure the aspect’s of pitching that a pitcher has the most control over. In 2011, the average FIP was 3.94 and the best FIP for starters was Roy Halladay at 2.20.
A note before I move on: FIP is often thought to be an ERA estimator, meaning it predicts a pitcher’s future ERA. This is not true (though it probably estimates future ERA better than ERA itself), as FIP tries to measure how well a pitcher has actually pitched, not how well he will pitch the in the future. Home run rates in particular are often not sustainable, although they are measured in FIP.
xFIP (Expected Fielding Independent Pitching): xFIP, unlike FIP, is an ERA predictor, so it projects a pitcher’s future ERA rather than measure past performance. xFIP is very similar to FIP in most respects, but instead of including home runs, it uses groundball rate to estimate how many home runs the pitcher should have given up given an average number of home runs per fly ball. It does this because studies have shown that home run per flyball ratios (HR/FB%) generally regress back to league average over time. xFIP is on the same scale as FIP (and ERA). The leader in xFIP last year was Zack Greinke at 2.56.
SIERA (Skill Interactive ERA): SIERA is similar to xFIP in that it predicts future ERA based on a small number of factors, but it is much more complicated to calculate, as it uses a lot of theories in order to predict ERA based on many statistical studies. All you need to know, however, is that it is one of the most accurate ERA predictors, sometimes more accurate than official projections. It is generally favorable towards pitchers with high strikeout rates, very high or very low groundball rates, and relievers. Last year, the leader in SIERA was again Zack Greinke at 2.66.
BABIP: I already explained what BABIP means above, but I want to make a quick note about BABIP for pitchers. Pitchers, for whatever reason, tend to have much less control over whether balls put in play against them fall for hits. Because of this, extreme BABIPs are much more likely to be out of the pitcher’s control. However, this does not mean that a pitcher’s BABIP will regress to the mean, because BABIP is largely based on the defense behind the pitcher. If his defenders can cover more ground and convert more balls into outs, then a pitcher’s BABIP will be lower, and vice versa.
Plate Discipline/SwStr%: Again, I will look at plate discipline stats for pitcher, which work basically the same as those for hitters. One very important one I will use is SwStr%, which is the percentage of all pitches that a batter swings and misses on. This is a very important stat for pitchers because it measures how well a pitcher is inducing swinging strikes. In turn, this correlates strongly with strikeout rate, so if a pitcher has a low SwStr% but a high strikeout rate, he may be due for regression.
PitchF/x: I’ll often use pitchf/x data, which measures the velocity and trajectory of every pitch. The data is extensive and confusing, but my main use of it will be to look at velocity, and the relative success of different types of pitches. For example, if a pitcher has thrown more curveballs this year, and is getting more swings and misses from those curveballs, I can see that information with pitchf/x.
Defense
UZR/150 (Ultimate Zone Rating per 150 games): Defense is one of the most difficult areas of baseball to quantify, and sabermetricians are still a long way away from finding a great stat to measure it. But UZR is one of the best metrics we have. Basically, it measures how many runs a fielder has saved relative to the average fielder at his position. It takes into account simple mistakes (errors) as well as range, arm, and other factors. The result is a number, negative or positive, that describes the number of runs the fielder has saved through defense.
UZR/150 turns UZR into a rate stat, measuring how many runs the player would save in 150 games played (given an average number of plays per game). This way we can compare the defense of players with difference amounts of playing time. Average UZR/150 is 0, and the league leader last year was Brett Gardner by a mile with a whopping 31 runs saved defensively per 150 games.
Other
WAR (Wins Above Replacement): WAR is one of the most famous sabermetrics stats, as it measures, simply, how many wins a player is/was worth over a player that a team can get for cheap from AAA or as a free agent. There are different ways to calculate WAR, and all of them have their flaws, but I will primarily use the one from FanGraphs.com, sometimes called fWAR. For batters, it is calculated using wOBA (see above), UZR (ditto), and a positional adjustment – that is, positions that are difficult to replace, such as catcher or shortstop, get a higher adjustment, and players from those positions will have a slightly higher WAR than others given equal offense and defense.
So there you have it. This post went much longer than I thought it would, and even so I’m sure I missed a bunch of stats that I’ll use in the upcoming series, but hopefully this is a helpful primer for those who are new to sabermetrics or those who just need a refresher. I’ll link back to this post often, so don’t worry if you forget what any of these terms mean. And if I ever refer to a stat that isn’t here or you would like more explanation about, please contact me and I’ll explain it further, or more likely send you links to more detailed and better explanations.
Most of these stats are taken from FanGraphs.com, and my explanations for them were largely influenced by their extensive glossary. If you are interested in learning more about sabermetric stats, I would strong suggest checking out FanGraphs’ glossary here.